Search inside The Magazine
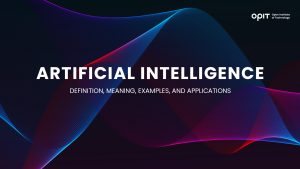
The artificial intelligence market was estimated to be worth $136 billion in 2022, with projections of up to $1,800 billion by the end of the decade. More than a third of companies today implement AI in their business processes, and over 40% will consider doing so in the future.
These whopping numbers testify to the importance, prevalence, and reality of AI in the modern world. If you’re considering an education in AI, you’re looking at a highly rewarding and prosperous future career. But what are the applications of artificial intelligence, and how did it all begin? Let’s start from scratch.
What Is Artificial Intelligence?
Artificial intelligence definition describes AI as a part of computer science that focuses on building programs and software with human intelligence. There are four types of artificial intelligence: the theory of mind, reactive, self-aware, and limited memory.
Reactive AI masters one field, like playing chess, performing a single manufacturing task, and similar. Limited memory machines can gather and remember information and use findings to offer recommendations (hotels, restaurants, etc.).
Theory of mind is a more developed type of AI capable of understanding human emotions. These machines can also take part in social interactions. Finally, self-aware AI is a conscious machine, but its development is reserved for the future.
History of Artificial Intelligence
The concept of artificial intelligence has roots in the 1950s. This was when AI became an academic discipline, and scientists started publishing papers about it. It all started with Alan Turing and his paper about computer machinery and intelligence that introduced basic AI concepts.
Here are some important milestones in the artificial intelligence field:
- 1952 – Arthur Samuel created a program that taught itself to play checkers.
- 1955 – John McCarthy’s workshop on AI, where the term was used for the first time.
- 1961 – First robot worker on a General Motors factory’s assembly line.
- 1980 – First conference on AI.
- 1986 – Demonstration of the first driverless car.
- 1997 – A program beat Gary Kasparov in a legendary chess match, thus becoming the first AI tool to win in a competition over a human.
- 2000 – Development of a robot that simulates a person’s body movement and human emotions.
AI in the 21st Century
The 21st century has witnessed some of the fastest advancements and applications of artificial intelligence across industries. Robots are becoming more sophisticated, they land on other planets, work in shops, clean, and much more. Global corporations like Facebook, Twitter, Netflix, and others regularly use AI tools in marketing to boost user experience, etc.
We’re also seeing the rise of AI chatbots like ChatGPT that can create content indistinguishable from human content.
Fields Used in Artificial Intelligence
Artificial intelligence relies on the use of numerous technologies:
- Machine Learning – Making apps and processes that can perform tasks like humans.
- Natural Language Processing – Training computers to understand words like humans.
- Computer Vision – Developing tools and programs that can read visual data and take information from it.
- Robotics – Programming agents to perform tasks in the physical world.
Applications of Artificial Intelligence
Below is an overview of applications of artificial intelligence across industries.
Automation
Any business and sector that relies on automation can use AI tools for faster data processing. By implementing advanced artificial intelligence tools into daily processes, you can save time and resources.
Healthcare
Fraud is common in healthcare. AI in this field is mostly oriented toward lowering the risk of fraud and administrative fees. For example, using AI makes it possible to check insurance claims and find inconsistencies.
Similarly, AI can help advance and finetune medical research, telemedicine, medical training, patient engagement, and support. There’s virtually no aspect of healthcare and medicine that couldn’t benefit from AI.
Business
Businesses across industries benefit from AI to finetune various aspects like the hiring process, threat detection, analytics, task automation, and more. Business owners and managers can make better-informed business decisions with less risk of error.
Education
Modern-day education offers personalized programs tailored to the individual learner’s abilities and goals. By automating tasks with AI tools, teachers can spend more time helping students progress faster in their studies.
Security
Security has never been more important following the rise of web applications, online shopping, and data sharing. With so much sensitive information shared daily, AI can help increase data protection and mitigate hacking attacks and threats. Systems with AI features can diagnose, scan, and detect threats.
Benefits and Challenges of Artificial Intelligence
There are enormous benefits of AI applications that can revolutionize any industry. Here are just some of them:
Automation and Increased Efficiency
AI helps streamline repetitive tasks, automate processes, and boost work efficiency. This characteristic of AI is already visible in all industries, and the use of programming languages like R and Python makes it all possible.
Improved Decision Making
Stakeholders can use AI to analyze immense amounts of data (with millions or billions of pieces of information) and make better-informed business decisions. Compare this to limited data analysis of the past, where researchers only had access to local documents or libraries, and you can understand how AI empowers present-day business owners.
Cost Savings
By automating tasks and streamlining processes, businesses also spend less money. Savings in terms of energy, extra work hour costs, materials, and even HR are significant. When you use AI right, you can turn almost any project into reality with minimal cost.
Challenges of AI
Despite the numerous benefits, AI also comes with a few challenges:
Data Privacy and Security
All AI developments take place online. The web still lacks proper laws on data protection and privacy, and it’s highly possible that user data is being used without consent in AI projects worldwide. Until strict laws are enacted, AI will continue to pose a threat to data privacy.
Algorithmic Bias
Algorithms today assist humans in decision-making. Stakeholders and regular users rely on data provided by AI tools to complete or approach tasks and even form new beliefs and behaviors. Poorly trained machines can encourage human biases, which can be especially harmful.
Job Less
AI is developing at the speed of light. Many tools are already replacing human labor in both the physical and digital worlds. A question remains to what degree machines will overtake the labor market in the future.
Artificial Intelligence Examples
Let’s look at real-world examples of artificial intelligence across applications and industries.
Virtual Assistants
Apple was the first company to introduce a virtual assistant based on AI. We know the tool today by the name of Siri. Numerous other companies like Amazon and Google have followed suit, so now we have Alexa, Google Assistant, and many other AI talking assistants.
Recommendation Systems
Users today find it ever more challenging to resist addictive content online. We’re often glued to our phones because our Instagram feed keeps suggesting must-watch Reels. The same goes for Netflix and its binge-worthy shows. These platforms use AI to enhance their recommendation system and offer ads, TV shows, or videos you love.
Shopping on Amazon works in a similar fashion. Even Spotify uses AI to offer audio recommendations to customers. It relies on your previous search history, liked content, and similar data to provide new suggestions.
Autonomous Vehicles
New-age vehicles powered by AI have sophisticated systems that make commuting easier than ever. Tesla’s latest AI software can collect information in real-time from the multiple cameras on the vehicles. The AI makes a 3D map with roads, obstacles, traffic lights, and other elements to make your ride safer.
Waymo has a similar system of lidar sensors around the vehicles that send pulsations around the car and offer an overview of the car’s surroundings.
Fraud Detection
Banks and credit card companies implement AI algorithms to prevent fraud. Advanced software helps these companies understand their customers and prevent non-authorized users from making payments or completing other unauthorized actions.
Image and Voice Recognition
If you have a newer smartphone, you’re already familiar with Face ID and voice assistant tools. These are built on basic AI principles and are being integrated into broader systems like vehicles, vending machines, home appliances, and more.
Deep Learning
Artificial intelligence encompasses both deep learning and machine learning. Machine learning encompasses deep learning and uses algorithms that learn from data, explore patterns, and predict outputs.
Deep learning relies on sophisticated neural networks similar to the networks in the human brain. Deep learning specialists use these neural networks to pinpoint patterns in large data sets.
Artificial Intelligence Continues to Grow and Develop
Although predicting the future is impossible, numerous AI specialists expect to see further development in this computer science discipline. More businesses will start implementing AI and we’ll see more autonomous vehicles and smarter robotics. That said, it’s increasingly important to take into account ethical considerations. As long as we use AI ethically, there’s no danger to our social interactions and privacy.
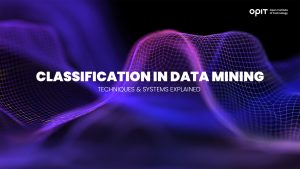
Data mining is an essential process for many businesses, including McDonald’s and Amazon. It involves analyzing huge chunks of unprocessed information to discover valuable insights. It’s no surprise large organizations rely on data mining, considering it helps them optimize customer service, reduce costs, and streamline their supply chain management.
Although it sounds simple, data mining is comprised of numerous procedures that help professionals extract useful information, one of which is classification. The role of this process is critical, as it allows data specialists to organize information for easier analysis.
This article will explore the importance of classification in greater detail. We’ll explain classification in data mining and the most common techniques.
Classification in Data Mining
Answering your question, “What is classification in data mining?” isn’t easy. To help you gain a better understanding of this term, we’ll cover the definition, purpose, and applications of classification in different industries.
Definition of Classification
Classification is the process of grouping related bits of information in a particular data set. Whether you’re dealing with a small or large set, you can utilize classification to organize the information more easily.
Purpose of Classification in Data Mining
Defining the classification of data mining systems is important, but why exactly do professionals use this method? The reason is simple – classification “declutters” a data set. It makes specific information easier to locate.
In this respect, think of classification as tidying up your bedroom. By organizing your clothes, shoes, electronics, and other items, you don’t have to waste time scouring the entire place to find them. They’re neatly organized and retrievable within seconds.
Applications of Classification in Various Industries
Here are some of the most common applications of data classification to help further demystify this process:
- Healthcare – Doctors can use data classification for numerous reasons. For example, they can group certain indicators of a disease for improved diagnostics. Likewise, classification comes in handy when grouping patients by age, condition, and other key factors.
- Finance – Data classification is essential for financial institutions. Banks can group information about consumers to find lenders more easily. Furthermore, data classification is crucial for elevating security.
- E-commerce – A key feature of online shopping platforms is recommending your next buy. They do so with the help of data classification. A system can analyze your previous decisions and group the related information to enhance recommendations.
- Weather forecast – Several considerations come into play during a weather forecast, including temperatures and humidity. Specialists can use a data mining platform to classify these considerations.
Techniques for Classification in Data Mining
Even though all data classification has a common goal (making information easily retrievable), there are different ways to accomplish it. In other words, you can incorporate an array of classification techniques in data mining.
Decision Trees
The decision tree method might be the most widely used classification technique. It’s a relatively simple yet effective method.
Overview of Decision Trees
Decision trees are like, well, trees, branching out in different directions. In the case of data mining, these trees have two branches: true and false. This method tells you whether a feature is true or false, allowing you to organize virtually any information.
Advantages and Disadvantages
Advantages:
- Preparing information in decision trees is simple.
- No normalization or scaling is involved.
- It’s easy to explain to non-technical staff.
Disadvantages:
- Even the tiniest of changes can transform the entire structure.
- Training decision tree-based models can be time-consuming.
- It can’t predict continuous values.
Support Vector Machines (SVM)
Another popular classification involves the use of support vector machines.
Overview of SVM
SVMs are algorithms that divide a dataset into two groups. It does so while ensuring there’s maximum distance from the margins of both groups. Once the algorithm categorizes information, it provides a clear boundary between the two groups.
Advantages and Disadvantages
Advantages:
- It requires minimal space.
- The process consumes little memory.
Disadvantages:
- It may not work well in large data sets.
- If the dataset has more features than training data samples, the algorithm might not be very accurate.
Naïve Bayes Classifier
The Naïve Bayes is also a viable option for classifying information.
Overview of Naïve Bayes Classifier
The Naïve Bayes method is a robust classification solution that makes predictions based on historical information. It tells you the likelihood of an event after analyzing how many times a similar (or the same) event has taken place. The most frequent application of this algorithm is distinguishing non-spam emails from billions of spam messages.
Advantages and Disadvantages
Advantages:
- It’s a fast, time-saving algorithm.
- Minimal training data is needed.
- It’s perfect for problems with multiple classes.
Disadvantages:
- Smoothing techniques are often required to fix noise.
- Estimates can be inaccurate.
K-Nearest Neighbors (KNN)
Although algorithms used for classification in data mining are complex, some have a simple premise. KNN is one of those algorithms.
Overview of KNN
Like many other algorithms, KNN starts with training data. From there, it determines the distance between particular objects. Items that are close to each other are considered related, which means that this system uses proximity to classify data.
Advantages and Disadvantages
Advantages:
- The implementation is simple.
- You can add new information whenever necessary without affecting the original data.
Disadvantages:
- The system can be computationally intensive, especially with large data sets.
- Calculating distances in large data sets is also expensive.
Artificial Neural Networks (ANN)
You might be wondering, “Is there a data classification technique that works like our brain?” Artificial neural networks may be the best example of such methods.
Overview of ANN
ANNs are like your brain. Just like the brain has connected neurons, ANNs have artificial neurons known as nodes that are linked to each other. Classification methods relying on this technique use the nodes to determine the category to which an object belongs.
Advantages and Disadvantages
Advantages:
- It can be perfect for generalization in natural language processing and image recognition since they can recognize patterns.
- The system works great for large data sets, as they render large chunks of information rapidly.
Disadvantages:
- It needs lots of training information and is expensive.
- The system can potentially identify non-existent patterns, which can make it inaccurate.
Comparison of Classification Techniques
It’s difficult to weigh up data classification techniques because there are significant differences. That’s not to say analyzing these models is like comparing apples to oranges. There are ways to determine which techniques outperform others when classifying particular information:
- ANNs generally work better than SVMs for making predictions.
- Decision trees are harder to design than some other, more complex solutions, such as ANNs.
- KNNs are typically more accurate than Naïve Bayes, which is rife with imprecise estimates.
Systems for Classification in Data Mining
Classifying information manually would be time-consuming. Thankfully, there are robust systems to help automate different classification techniques in data mining.
Overview of Data Mining Systems
Data mining systems are platforms that utilize various methods of classification in data mining to categorize data. These tools are highly convenient, as they speed up the classification process and have a multitude of applications across industries.
Popular Data Mining Systems for Classification
Like any other technology, classification of data mining systems becomes easier if you use top-rated tools:
WEKA
How often do you need to add algorithms from your Java environment to classify a data set? If you do it regularly, you should use a tool specifically designed for this task – WEKA. It’s a collection of algorithms that performs a host of data mining projects. You can apply the algorithms to your own code or directly into the platform.
RapidMiner
If speed is a priority, consider integrating RapidMiner into your environment. It produces highly accurate predictions in double-quick time using deep learning and other advanced techniques in its Java-based architecture.
Orange
Open-source platforms are popular, and it’s easy to see why when you consider Orange. It’s an open-source program with powerful classification and visualization tools.
KNIME
KNIME is another open-source tool you can consider. It can help you classify data by revealing hidden patterns in large amounts of information.
Apache Mahout
Apache Mahout allows you to create algorithms of your own. Each algorithm developed is scalable, enabling you to transfer your classification techniques to higher levels.
Factors to Consider When Choosing a Data Mining System
Choosing a data mining system is like buying a car. You need to ensure the product has particular features to make an informed decision:
- Data classification techniques
- Visualization tools
- Scalability
- Potential issues
- Data types
The Future of Classification in Data Mining
No data mining discussion would be complete without looking at future applications.
Emerging Trends in Classification Techniques
Here are the most important data classification facts to keep in mind for the foreseeable future:
- The amount of data should rise to 175 billion terabytes by 2025.
- Some governments may lift certain restrictions on data sharing.
- Data automation is expected to be further automated.
Integration of Classification With Other Data Mining Tasks
Classification is already an essential task. Future platforms may combine it with clustering, regression, sequential patterns, and other techniques to optimize the process. More specifically, experts may use classification to better organize data for subsequent data mining efforts.
The Role of Artificial Intelligence and Machine Learning in Classification
Nearly 20% of analysts predict machine learning and artificial intelligence will spearhead the development of classification strategies. Hence, mastering these two technologies may become essential.
Data Knowledge Declassified
Various methods for data classification in data mining, like decision trees and ANNs, are a must-have in today’s tech-driven world. They help healthcare professionals, banks, and other industry experts organize information more easily and make predictions.
To explore this data mining topic in greater detail, consider taking a course at an accredited institution. You’ll learn the ins and outs of data classification as well as expand your career options.
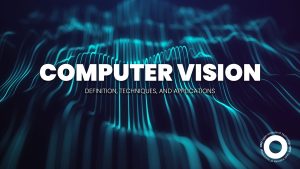
For most people, identifying objects surrounding them is an easy task.
Let’s say you’re in your office. You can probably casually list objects like desks, computers, filing cabinets, printers, and so on. While this action seems simple on the surface, human vision is actually quite complex.
So, it’s not surprising that computer vision – a relatively new branch of technology aiming to replicate human vision – is equally, if not more, complex.
But before we dive into these complexities, let’s understand the basics – what is computer vision?
Computer vision is an artificial intelligence (AI) field focused on enabling computers to identify and process objects in the visual world. This technology also equips computers to take action and make recommendations based on the visual input they receive.
Simply put, computer vision enables machines to see and understand.
Learning the computer vision definition is just the beginning of understanding this fascinating field. So, let’s explore the ins and outs of computer vision, from fundamental principles to future trends.
History of Computer Vision
While major breakthroughs in computer vision have occurred relatively recently, scientists have been training machines to “see” for over 60 years.
To do the math – the research on computer vision started in the late 1950s.
Interestingly, one of the earliest test subjects wasn’t a computer. Instead, it was a cat! Scientists used a little feline helper to examine how their nerve cells respond to various images. Thanks to this experiment, they concluded that detecting simple shapes is the first stage in image processing.
As AI emerged as an academic field of study in the 1960s, a decade-long quest to help machines mimic human vision officially began.
Since then, there have been several significant milestones in computer vision, AI, and deep learning. Here’s a quick rundown for you:
- 1970s – Computer vision was used commercially for the first time to help interpret written text for the visually impaired.
- 1980s – Scientists developed convolutional neural networks (CNNs), a key component in computer vision and image processing.
- 1990s – Facial recognition tools became highly popular, thanks to a shiny new thing called the internet. For the first time, large sets of images became available online.
- 2000s – Tagging and annotating visual data sets were standardized.
- 2010s – Alex Krizhevsky developed a CNN model called AlexNet, drastically reducing the error rate in image recognition (and winning an international image recognition contest in the process).
Today, computer vision algorithms and techniques are rapidly developing and improving. They owe this to an unprecedented amount of visual data and more powerful hardware.
Thanks to these advancements, 99% accuracy has been achieved for computer vision, meaning it’s currently more accurate than human vision at quickly identifying visual inputs.
Fundamentals of Computer Vision
New functionalities are constantly added to the computer vision systems being developed. Still, this doesn’t take away from the same fundamental functions these systems share.
Image Acquisition and Processing
Without visual input, there would be no computer vision. So, let’s start at the beginning.
The image acquisition function first asks the following question: “What imaging device is used to produce the digital image?”
Depending on the device, the resulting data can be a 2D, 3D image, or an image sequence. These images are then processed, allowing the machine to verify whether the visual input contains satisfying data.
Feature Extraction and Representation
The next question then becomes, “What specific features can be extracted from the image?”
By features, we mean measurable pieces of data unique to specific objects in the image.
Feature extraction focuses on extracting lines and edges and localizing interest points like corners and blobs. To successfully extract these features, the machine breaks the initial data set into more manageable chunks.
Object Recognition and Classification
Next, the computer vision system aims to answer: “What objects or object categories are present in the image, and where are they?”
This interpretive technique recognizes and classifies objects based on large amounts of pre-learned objects and object categories.
Image Segmentation and Scene Understanding
Besides observing what is in the image, today’s computer vision systems can act based on those observations.
In image segmentation, computer vision algorithms divide the image into multiple regions and examine the relevant regions separately. This allows them to gain a full understanding of the scene, including the spatial and functional relationships between the present objects.
Motion Analysis and Tracking
Motion analysis studies movements in a sequence of digital images. This technique correlates to motion tracking, which follows the movement of objects of interest. Both techniques are commonly used in manufacturing for monitoring machinery.
Key Techniques and Algorithms in Computer Vision
Computer vision is a fairly complex task. For starters, it needs a huge amount of data. Once the data is all there, the system runs multiple analyses to achieve image recognition.
This might sound simple, but this process isn’t exactly straightforward.
Think of computer vision as a detective solving a crime. What does the detective need to do to identify the criminal? Piece together various clues.
Similarly (albeit with less danger), a computer vision model relies on colors, shapes, and patterns to piece together an object and identify its features.
Let’s discuss the techniques and algorithms this model uses to achieve its end result.
Convolutional Neural Networks (CNNs)
In computer vision, CNNs extract patterns and employ mathematical operations to estimate what image they’re seeing. And that’s all there really is to it. They continue performing the same mathematical operation until they verify the accuracy of their estimate.
Deep Learning and Transfer Learning
The advent of deep learning removed many constraints that prevented computer vision from being widely used. On top of that, (and luckily for computer scientists!), it also eliminated all the tedious manual work.
Essentially, deep learning enables a computer to learn about visual data independently. Computer scientists only need to develop a good algorithm, and the machine will take care of the rest.
Alternatively, computer vision can use a pre-trained model as a starting point. This concept is known as transfer learning.
Edge Detection and Feature Extraction Techniques
Edge detection is one of the most prominent feature extraction techniques.
As the name suggests, it can identify the boundaries of an object and extract its features. As always, the ultimate goal is identifying the object in the picture. To achieve this, edge detection uses an algorithm that identifies differences in pixel brightness (after transforming the data into a grayscale image).
Optical Flow and Motion Estimation
Optical flow is a computer vision technique that determines how each point of an image or video sequence is moving compared to the image plane. This technique can estimate how fast objects are moving.
Motion estimation, on the other hand, predicts the location of objects in subsequent frames of a video sequence.
These techniques are used in object tracking and autonomous navigation.
Image Registration and Stitching
Image registration and stitching are computer vision techniques used to combine multiple images. Image registration is responsible for aligning these images, while image stitching overlaps them to produce a single image. Medical professionals use these techniques to track the progress of a disease.
Applications of Computer Vision
Thanks to many technological advances in the field, computer vision has managed to surpass human vision in several regards. As a result, it’s used in various applications across multiple industries.
Robotics and Automation
Improving robotics was one of the original reasons for developing computer vision. So, it isn’t surprising this technique is used extensively in robotics and automation.
Computer vision can be used to:
- Control and automate industrial processes
- Perform automatic inspections in manufacturing applications
- Identify product and machine defects in real time
- Operate autonomous vehicles
- Operate drones (and capture aerial imaging)
Security and Surveillance
Computer vision has numerous applications in video surveillance, including:
- Facial recognition for identification purposes
- Anomaly detection for spotting unusual patterns
- People counting for retail analytics
- Crowd monitoring for public safety
Healthcare and Medical Imaging
Healthcare is one of the most prominent fields of computer vision applications. Here, this technology is employed to:
- Establish more accurate disease diagnoses
- Analyze MRI, CAT, and X-ray scans
- Enhance medical images interpreted by humans
- Assist surgeons during surgery
Entertainment and Gaming
Computer vision techniques are highly useful in the entertainment industry, supporting the creation of visual effects and motion capture for animation.
Good news for gamers, too – computer vision aids augmented and virtual reality in creating the ultimate gaming experience.
Retail and E-Commerce
Self-check-out points can significantly enhance the shopping experience. And guess what can help establish them? That’s right – computer vision. But that’s not all. This technology also helps retailers with inventory management, allowing quicker detection of out-of-stock products.
In e-commerce, computer vision facilitates visual search and product recommendation, streamlining the (often frustrating) online purchasing process.
Challenges and Limitations of Computer Vision
There’s no doubt computer vision has experienced some major breakthroughs in recent years. Still, no technology is without flaws.
Here are some of the challenges that computer scientists hope to overcome in the near future:
- The data for training computer vision models often lack in quantity or quality.
- There’s a need for more specialists who can train and monitor computer vision models.
- Computers still struggle to process incomplete, distorted, and previously unseen visual data.
- Building computer vision systems is still complex, time-consuming, and costly.
- Many people have privacy and ethical concerns surrounding computer vision, especially for surveillance.
Future Trends and Developments in Computer Vision
As the field of computer vision continues to develop, there should be no shortage of changes and improvements.
These include integration with other AI technologies (such as neuro-symbolic and explainable AI), which will continue to evolve as developing hardware adds new capabilities and capacities that enhance computer vision. Each advancement brings with it the opportunity for other industries (and more complex applications). Construction gives us a good example, as computer vision takes us away from the days of relying on hard hats and signage, moving us toward a future in which computers can actively detect, and alert site foremen too, unsafe behavior.
The Future Looks Bright for Computer Vision
Computer vision is one of the most remarkable concepts in the world of deep learning and artificial intelligence. This field will undoubtedly continue to grow at an impressive speed, both in terms of research and applications.
Are you interested in further research and professional development in this field? If yes, consider seeking out high-quality education in computer vision.
Have questions?
Visit our FAQ page or get in touch with us!
Write us at +39 335 576 0263
Get in touch at hello@opit.com
Talk to one of our Study Advisors
We are international
We can speak in: