Zorina Alliata Of Open Institute of Technology On Five Things You Need To Create A Highly Successful Career In The AI Industry
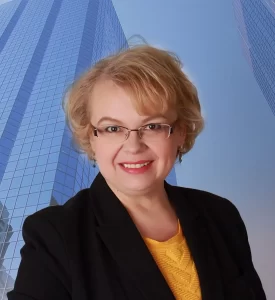
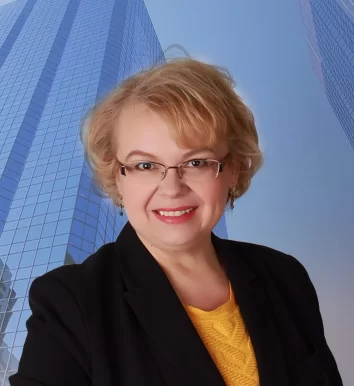
Source:
- Authority Magazine Medium, Published on September 15th, 2024.
Gaining hands-on experience through projects, internships, and collaborations is vital for understanding how to apply AI in various industries and domains. Use Kaggle or get a free cloud account and start experimenting. You will have projects to discuss at your next interviews.
By David Leichner, CMO at Cybellum
14 min read
Artificial Intelligence is now the leading edge of technology, driving unprecedented advancements across sectors. From healthcare to finance, education to environment, the AI industry is witnessing a skyrocketing demand for professionals. However, the path to creating a successful career in AI is multifaceted and constantly evolving. What does it take and what does one need in order to create a highly successful career in AI?
In this interview series, we are talking to successful AI professionals, AI founders, AI CEOs, educators in the field, AI researchers, HR managers in tech companies, and anyone who holds authority in the realm of Artificial Intelligence to inspire and guide those who are eager to embark on this exciting career path.
As part of this series, we had the pleasure of interviewing Zorina Alliata.
Zorina Alliata is an expert in AI, with over 20 years of experience in tech, and over 10 years in AI itself. As an educator, Zorina Alliata is passionate about learning, access to education and about creating the career you want. She implores us to learn more about ethics in AI, and not to fear AI, but to embrace it.
Thank you so much for joining us in this interview series! Before we dive in, our readers would like to learn a bit about your origin story. Can you share with us a bit about your childhood and how you grew up?
I was born in Romania, and grew up during communism, a very dark period in our history. I was a curious child and my parents, both teachers, encouraged me to learn new things all the time. Unfortunately, in communism, there was not a lot to do for a kid who wanted to learn: there was no TV, very few books and only ones that were approved by the state, and generally very few activities outside of school. Being an “intellectual” was a bad thing in the eyes of the government. They preferred people who did not read or think too much. I found great relief in writing, I have been writing stories and poetry since I was about ten years old. I was published with my first poem at 16 years old, in a national literature magazine.
Can you share with us the ‘backstory’ of how you decided to pursue a career path in AI?
I studied Computer Science at university. By then, communism had fallen and we actually had received brand new PCs at the university, and learned several programming languages. The last year, the fifth year of study, was equivalent with a Master’s degree, and was spent preparing your thesis. That’s when I learned about neural networks. We had a tiny, 5-node neural network and we spent the year trying to teach it to recognize the written letter “A”.
We had only a few computers in the lab running Windows NT, so really the technology was not there for such an ambitious project. We did not achieve a lot that year, but I was fascinated by the idea of a neural network learning by itself, without any programming. When I graduated, there were no jobs in AI at all, it was what we now call “the AI winter”. So I went and worked as a programmer, then moved into management and project management. You can imagine my happiness when, about ten years ago, AI came back to life in the form of Machine Learning (ML).
I immediately went and took every class possible to learn about it. I spent that Christmas holiday coding. The paradigm had changed from when I was in college, when we were trying to replicate the entire human brain. ML was focused on solving one specific problem, optimizing one specific output, and that’s where businesses everywhere saw a benefit. I then joined a Data Science team at GEICO, moved to Capital One as a Delivery lead for their Center for Machine Learning, and then went to Amazon in their AI/ML team.
Can you tell our readers about the most interesting projects you are working on now?
While I can’t discuss work projects due to confidentiality, there are some things I can mention! In the last five years, I worked with global companies to establish an AI strategy and to introduce AI and ML in their organizations. Some of my customers included large farming associations, who used ML to predict when to plant their crops for optimal results; water management companies who used ML for predictive maintenance to maintain their underground pipes; construction companies that used AI for visual inspections of their buildings, and to identify any possible defects and hospitals who used Digital Twins technology to improve patient outcomes and health. It is amazing to see how much AI and ML are already part of our everyday lives, and to recognize some of it in the mundane around us.
None of us are able to achieve success without some help along the way. Is there a particular person who you are grateful for who helped get you to where you are? Can you share a story about that?
When you are young, there are so many people who step up and help you along the way. I have had great luck with several professors who have encouraged me in school, and an uncle who worked in computers who would take me to his office and let me play around with his machines. I now try to give back and mentor several young people, especially women who are trying to get into the field. I volunteer with AnitaB and Zonta, as well as taking on mentees where I work.
As with any career path, the AI industry comes with its own set of challenges. Could you elaborate on some of the significant challenges you faced in your AI career and how you managed to overcome them?
I think one major challenge in AI is the speed of change. I remember after spending my Christmas holiday learning and coding in R, when I joined the Data Science team at GEICO, I realized the world had moved on and everyone was now coding in Python. So, I had to learn Python very fast, in order to understand what was going on.
It’s the same with research — I try to work on one subject, and four new papers are published every week that move the goal posts. It is very challenging to keep up, but you just have to adapt to continuously learn and let go of what becomes obsolete.
Ok, let’s now move to the main part of our interview about AI. What are the 3 things that most excite you about the AI industry now? Why?
1. Creativity
Generative AI brought us the ability to create amazing images based on simple text descriptions. Entire videos are now possible, and soon, maybe entire movies. I have been working in AI for several years and I never thought creative jobs will be the first to be achieved by AI. I am amazed at the capacity of an algorithms to create images, and to observe the artificial creativity we now see for the first time.
2. Abstraction
I think with the success and immediate mainstream adoption of Generative AI, we saw the great appetite out there for automation and abstraction. No one wants to do boring work and summarizing documents; no one wants to read long websites, they just want the gist of it. If I drive a car, I don’t need to know how the engine works and every equation that the engineers used to build it — I just want my car to drive. The same level of abstraction is now expected in AI. There is a lot of opportunity here in creating these abstractions for the future.
3. Opportunity
I like that we are in the beginning of AI, so there is a lot of opportunity to jump in. Most people who are passionate about it can learn all about AI fully online, in places like Open Institute of Technology. Or they can get experience working on small projects, and then they can apply for jobs. It is great because it gives people access to good jobs and stability in the future.
What are the 3 things that concern you about the AI industry? Why? What should be done to address and alleviate those concerns?
1. Fairness
The large companies that build LLMs spend a lot of energy and money into making them fair. But it is not easy. Us, as humans, are often not fair ourselves. We even have problems agreeing what fairness even means. So, how can we teach the machines to be fair? I think the responsibility stays with us. We can’t simply say “AI did this bad thing.”
2. Regulation
There are some regulations popping up but most are not coordinated or discussed widely. There is controversy, such as regarding the new California bill SB1047, where scientists take different sides of the debate. We need to find better ways to regulate the use and creation of AI, working together as a society, not just in small groups of politicians.
3. Awareness
I wish everyone understood the basics of AI. There is denial, fear, hatred that is created by doomsday misinformation. I wish AI was taught from a young age, through appropriate means, so everyone gets the fundamental principles and understands how to use this great tool in their lives.
For a young person who would like to eventually make a career in AI, which skills and subjects do they need to learn?
I think maybe the right question is: what are you passionate about? Do that, and see how you can use AI to make your job better and more exciting! I think AI will work alongside people in most jobs, as it develops and matures.
But for those who are looking to work in AI, they can choose from a variety of roles as well. We have technical roles like data scientist or machine learning engineer, which require very specialized knowledge and degrees. They learn computing, software engineering, programming, data analysis, data engineering. There are also business roles, for people who understand the technology well but are not writing code. Instead, they define strategies, design solutions for companies, or write implementation plans for AI products and services. There is also a robust AI research domain, where lots of scientists are measuring and analyzing new technology developments.
With Generative AI, new roles appeared, such as Prompt Engineer. We can now talk with the machines in natural language, so speaking good English is all that’s required to find the right conversation.
With these many possible roles, I think if you work in AI, some basic subjects where you can start are:
- Analytics — understand data and how it is stored and governed, and how we get insights from it.
- Logic — understand both mathematical and philosophical logic.
- Fundamentals of AI — read about the history and philosophy of AI, models of thinking, and major developments.
As you know, there are not that many women in the AI industry. Can you advise what is needed to engage more women in the AI industry?
Engaging more women in the AI industry is absolutely crucial if you want to build any successful AI products. In my twenty years career, I have seen changes in the tech industry to address this gender discrepancy. For example, we do well in school with STEM programs and similar efforts that encourage girls to code. We also created mentorship organizations such as AnitaB.org who allow women to connect and collaborate. One place where I think we still lag behind is in the workplace. When I came to the US in my twenties, I was the only woman programmer in my team. Now, I see more women at work, but still not enough. We say we create inclusive work environments, but we still have a long way to go to encourage more women to stay in tech. Policies that support flexible hours and parental leave are necessary, and other adjustments that account for the different lives that women have compared to men. Bias training and challenging stereotypes are also necessary, and many times these are implemented shoddily in organizations.
Ethical AI development is a pressing concern in the industry. How do you approach the ethical implications of AI, and what steps do you believe individuals and organizations should take to ensure responsible and fair AI practices?
Machine Learning and AI learn from data. Unfortunately, lot of our historical data shows strong biases. For example, for a long time, it was perfectly legal to only offer mortgages to white people. The data shows that. If we use this data to train a new model to enhance the mortgage application process, then the model will learn that mortgages should only be offered to white men. That is a bias that we had in the past, but we do not want to learn and amplify in the future.
Generative AI has introduced a new set of fresh risks, the most famous being the “hallucinations.” Generative AI will create new content based on chunks of text it finds in its training data, without an understanding of what the content means. It could repeat something it learned from one Reddit user ten years ago, that could be factually incorrect. Is that piece of information unbiased and fair?
There are many ways we fight for fairness in AI. There are technical tools we can use to offer interpretability and explainability of the actual models used. There are business constraints we can create, such as guardrails or knowledge bases, where we can lead the AI towards ethical answers. We also advise anyone who build AI to use a diverse team of builders. If you look around the table and you see the same type of guys who went to the schools, you will get exactly one original idea from them. If you add different genders, different ages, different tenures, different backgrounds, then you will get ten innovative ideas for your product, and you will have addressed biases you’ve never even thought of.
Read the full article below:
Have questions?
Visit our FAQ page or get in touch with us!
Write us at +39 335 576 0263
Get in touch at hello@opit.com
Talk to one of our Study Advisors
We are international
We can speak in: