Data Science & AI
Dive deep into data-driven technologies: Machine Learning, Reinforcement Learning, Data Mining, Big Data, NLP & more. Stay updated.
Search inside The Magazine
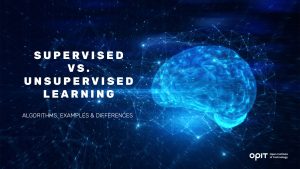
The human brain is among the most complicated organs and one of nature’s most amazing creations. The brain’s capacity is considered limitless; there isn’t a thing it can’t remember. Although many often don’t think about it, the processes that happen in the mind are fascinating.
As technology evolved over the years, scientists figured out a way to make machines think like humans, and this process is called machine learning. Like cars need fuel to operate, machines need data and algorithms. With the application of adequate techniques, machines can learn from this data and even improve their accuracy as time passes.
Two basic machine learning approaches are supervised and unsupervised learning. You can already assume the biggest difference between them based on their names. With supervised learning, you have a “teacher” who shows the machine how to analyze specific data. Unsupervised learning is completely independent, meaning there are no teachers or guides.
This article will talk more about supervised and unsupervised learning, outline their differences, and introduce examples.
Supervised Learning
Imagine a teacher trying to teach their young students to write the letter “A.” The teacher will first set an example by writing the letter on the board, and the students will follow. After some time, the students will be able to write the letter without assistance.
Supervised machine learning is very similar to this situation. In this case, you (the teacher) train the machine using labeled data. Such data already contains the right answer to a particular situation. The machine then uses this training data to learn a pattern and applies it to all new datasets.
Note that the role of a teacher is essential. The provided labeled datasets are the foundation of the machine’s learning process. If you withhold these datasets or don’t label them correctly, you won’t get any (relevant) results.
Supervised learning is complex, but we can understand it through a simple real-life example.
Suppose you have a basket filled with red apples, strawberries, and pears and want to train a machine to identify these fruits. You’ll teach the machine the basic characteristics of each fruit found in the basket, focusing on the color, size, shape, and other relevant features. If you introduce a “new” strawberry to the basket, the machine will analyze its appearance and label it as “strawberry” based on the knowledge it acquired during training.
Types of Supervised Learning
You can divide supervised learning into two types:
- Classification – You can train machines to classify data into categories based on different characteristics. The fruit basket example is the perfect representation of this scenario.
- Regression – You can train machines to use specific data to make future predictions and identify trends.
Supervised Learning Algorithms
Supervised learning uses different algorithms to function:
- Linear regression – It identifies a linear relationship between an independent and a dependent variable.
- Logistic regression – It typically predicts binary outcomes (yes/no, true/false) and is important for classification purposes.
- Support vector machines – They use high-dimensional features to map data that can’t be separated by a linear line.
- Decision trees – They predict outcomes and classify data using tree-like structures.
- Random forests – They analyze several decision trees to come up with a unique prediction/result.
- Neural networks – They process data in a unique way, very similar to the human brain.
Supervised Learning: Examples and Applications
There’s no better way to understand supervised learning than through examples. Let’s dive into the real estate world.
Suppose you’re a real estate agent and need to predict the prices of different properties in your city. The first thing you’ll need to do is feed your machine existing data about available houses in the area. Factors like square footage, amenities, a backyard/garden, the number of rooms, and available furniture, are all relevant factors. Then, you need to “teach” the machine the prices of different properties. The more, the better.
A large dataset will help your machine pick up on seemingly minor but significant trends affecting the price. Once your machine processes this data and you introduce a new property to it, it will be able to cross-reference its features with the existing database and come up with an accurate price prediction.
The applications of supervised learning are vast. Here are the most popular ones:
- Sales – Predicting customers’ purchasing behavior and trends
- Finance – Predicting stock market fluctuations, price changes, expenses, etc.
- Healthcare – Predicting risk of diseases and infections, surgery outcomes, necessary medications, etc.
- Weather forecasts – Predicting temperature, humidity, atmospheric pressure, wind speed, etc.
- Face recognition – Identifying people in photos
Unsupervised Learning
Imagine a family with a baby and a dog. The dog lives inside the house, so the baby is used to it and expresses positive emotions toward it. A month later, a friend comes to visit, and they bring their dog. The baby hasn’t seen the dog before, but she starts smiling as soon as she sees it.
Why?
Because the baby was able to draw her own conclusions based on the new dog’s appearance: two ears, tail, nose, tongue sticking out, and maybe even a specific noise (barking). Since the baby has positive emotions toward the house dog, she also reacts positively to a new, unknown dog.
This is a real-life example of unsupervised learning. Nobody taught the baby about dogs, but she still managed to make accurate conclusions.
With supervised machine learning, you have a teacher who trains the machine. This isn’t the case with unsupervised learning. Here, it’s necessary to give the machine freedom to explore and discover information. Therefore, this machine learning approach deals with unlabeled data.
Types of Unsupervised Learning
There are two types of unsupervised learning:
- Clustering – Grouping uncategorized data based on their common features.
- Dimensionality reduction – Reducing the number of variables, features, or columns to capture the essence of the available information.
Unsupervised Learning Algorithms
Unsupervised learning relies on these algorithms:
- K-means clustering – It identifies similar features and groups them into clusters.
- Hierarchical clustering – It identifies similarities and differences between data and groups them hierarchically.
- Principal component analysis (PCA) – It reduces data dimensionality while boosting interpretability.
- Independent component analysis (ICA) – It separates independent sources from mixed signals.
- T-distributed stochastic neighbor embedding (t-SNE) – It explores and visualizes high-dimensional data.
Unsupervised Learning: Examples and Applications
Let’s see how unsupervised learning is used in customer segmentation.
Suppose you work for a company that wants to learn more about its customers to build more effective marketing campaigns and sell more products. You can use unsupervised machine learning to analyze characteristics like gender, age, education, location, and income. This approach is able to discover who purchases your products more often. After getting the results, you can come up with strategies to push the product more.
Unsupervised learning is often used in the same industries as supervised learning but with different purposes. For example, both approaches are used in sales. Supervised learning can accurately predict prices relying on past data. On the other hand, unsupervised learning analyzes the customers’ behaviors. The combination of the two approaches results in a quality marketing strategy that can attract more buyers and boost sales.
Another example is traffic. Supervised learning can provide an ETA to a destination, while unsupervised learning digs a bit deeper and often looks at the bigger picture. It can analyze a specific area to pinpoint accident-prone locations.
Differences Between Supervised and Unsupervised Learning
These are the crucial differences between the two machine learning approaches:
- Data labeling – Supervised learning uses labeled datasets, while unsupervised learning uses unlabeled, “raw” data. In other words, the former requires training, while the latter works independently to discover information.
- Algorithm complexity – Unsupervised learning requires more complex algorithms and powerful tools that can handle vast amounts of data. This is both a drawback and an advantage. Since it operates on complex algorithms, it’s capable of handling larger, more complicated datasets, which isn’t a characteristic of supervised learning.
- Use cases and applications – The two approaches can be used in the same industries but with different purposes. For example, supervised learning is used in predicting prices, while unsupervised learning is used in detecting customers’ behavior or anomalies.
- Evaluation metrics – Supervised learning tends to be more accurate (at least for now). Machines still require a bit of our input to display accurate results.
Choose Wisely
Do you need to teach your machine different data, or can you trust it to handle the analysis on its own? Think about what you want to analyze. Unsupervised and supervised learning may sound similar, but they have different uses. Choosing an inadequate approach leads to unreliable, irrelevant results.
Supervised learning is still more popular than unsupervised learning because it offers more accurate results. However, this approach can’t handle larger, complex datasets and requires human intervention, which isn’t the case with unsupervised learning. Therefore, we may see a rise in the popularity of the unsupervised approach, especially as the technology evolves and enables more accuracy.
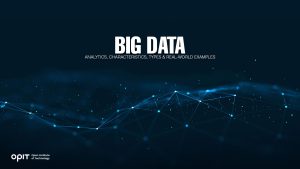
The term “big data” is self-explanatory: it’s a large collection of data. However, to be classified as “big,” data needs to meet specific criteria. Big data is huge in volume, gets even bigger over time, arrives with ever-higher velocity, and is so complex that no traditional tools can handle it.
Big data analytics is the (complex) process of analyzing these huge chunks of data to discover different information. The process is especially important for small companies that use the uncovered information to design marketing strategies, conduct market research, and follow the latest industry trends.
In this introduction to big data analytics, we’ll dig deep into big data and uncover ways to analyze it. We’ll also explore its (relatively short) history and evolution and present its advantages and drawbacks.
History and Evolution of Big Data
We’ll start this introduction to big data with a short history lesson. After all, we can’t fully answer the “what is big data?” question if we don’t know its origins.
Let’s turn on our time machine and go back to the 1960s. That’s when the first major change that marked the beginning of the big data era took place. The advanced development of data centers, databases, and innovative processing methods facilitated the rise of big data.
Relational databases (storing and offering access to interconnected data points) have become increasingly popular. While people had ways to store data much earlier, experts consider that this decade set the foundations for the development of big data.
The next major milestone was the emergence of the internet and the exponential growth of data. This incredible invention made handling and analyzing large chunks of information possible. As the internet developed, big data technologies and tools became more advanced.
This leads us to the final destination of short time travel: the development of big data analytics, i.e., processes that allow us to “digest” big data. Since we’re witnessing exceptional technological developments, the big data journey is yet to continue. We can only expect the industry to advance further and offer more options.
Big Data Technologies and Tools
What tools and technologies are used to decipher big data and offer value?
Data Storage and Management
Data storage and management tools are like virtual warehouses where you can pack up your big data safely and work with it as needed. These tools feature a powerful infrastructure that lets you access and fetch the desired information quickly and easily.
Data Processing and Analytics Framework
Processing and analyzing huge amounts of data are no walk in the park. But they can be, thanks to specific tools and technologies. These valuable allies can clean and transform large piles of information into data you can use to pursue your goals.
Machine Learning and Artificial Intelligence Platforms
Machine learning and artificial intelligence platforms “eat” big data and perform a wide array of functions based on the discoveries. These technologies can come in handy with testing hypotheses and making important decisions. Best of all, they require minimal human input; you can relax while AI works its magic.
Data Visualization Tools
Making sense of large amounts of data and presenting it to investors, stakeholders, and team members can feel like a nightmare. Fortunately, you can turn this nightmare into a dream come true with big data visualization tools. Thanks to the tools, creating stunning graphs, dashboards, charts, and tables and impressing your coworkers and superiors has never been easier.
Big Data Analytics Techniques and Methods
What techniques and methods are used in big data analytics? Let’s find the answer.
Descriptive Analytics
Descriptive analytics is like a magic wand that turns raw data into something people can read and understand. Whether you want to generate reports, present data on a company’s revenue, or analyze social media metrics, descriptive analytics is the way to go.
It’s mostly used for:
- Data summarization and aggregation
- Data visualization
Diagnostic Analytics
Have a problem and want to get detailed insight into it? Diagnostic analytics can help. It identifies the root of an issue, helping you figure out your next move.
Some methods used in diagnostic analytics are:
- Data mining
- Root cause analysis
Predictive Analytics
Predictive analytics is like a psychic that looks into the future to predict different trends.
Predictive analytics often uses:
- Regression analysis
- Time series analysis
Prescriptive Analytics
Prescriptive analytics is an almighty problem-solver. It usually joins forces with descriptive and predictive analytics to offer an ideal solution to a particular problem.
Some methods prescriptive analytics uses are:
- Optimization techniques
- Simulation and modeling
Applications of Big Data Analytics
Big data analytics has found its home in many industries. It’s like the not-so-secret ingredient that can make the most of any niche and lead to desired results.
Business and Finance
How do business and finance benefit from big data analytics? These industries can flourish through better decision-making, investment planning, fraud detection and prevention, and customer segmentation and targeting.
Healthcare
Healthcare is another industry that benefits from big data analytics. In healthcare, big data is used to create patient databases, personal treatment plans, and electronic health records. This data also serves as an excellent foundation for accurate statistics about treatments, diseases, patient backgrounds, risk factors, etc.
Government and Public Sector
Big data analytics has an important role in government and the public sector. Analyzing different data improves efficiency in terms of costs, innovation, crime prediction and prevention, and workforce. Multiple government parts often need to work together to get the best results.
As technology advances, big data analytics has found another major use in the government and public sector: smart cities and infrastructure. With precise and thorough analysis, it’s possible to bring innovation and progress and implement the latest features and digital solutions.
Sports and Entertainment
Sports and entertainment are all about analyzing the past to predict the future and improve performance. Whether it’s analyzing players to create winning strategies or attracting the audience and freshening up the content, big data analytics is like a valuable player everyone wants on their team.
Challenges and Ethical Considerations in Big Data Analytics
Big data analytics represent doors to new worlds of information. But opening these doors often comes with certain challenges and ethical considerations.
Data Privacy and Security
One of the major challenges (and the reason some people aren’t fans of big data analytics) is data privacy and security. The mere fact that personal information can be used in big data analytics can make individuals feel exploited. Since data breaches and identity thefts are, unfortunately, becoming more common, it’s no surprise some people feel this way.
Fortunately, laws like GDPR and CCPA give individuals more control over the information others can collect from them.
Data Quality and Accuracy
Big data analytics can sometimes be a dead end. If the material wasn’t handled correctly, or the data was incomplete to start with, the results themselves won’t be adequate.
Algorithmic Bias and Fairness
Big data analytics is based on algorithms, which are designed by humans. Hence, it’s not unusual to assume that these algorithms can be biased (or unfair) due to human prejudices.
Ethical Use of Big Data Analytics
The ethical use of big data analytics concerns the “right” and “wrong” in terms of data usage. Can big data’s potential be exploited to the fullest without affecting people’s right to privacy?
Future Trends and Opportunities in Big Data Analytics
Although it has proven useful in many industries, big data analytics is still relatively young and unexplored.
Integration of Big Data Analytics With Emerging Technologies
It seems that new technologies appear in the blink of an eye. Our reality today (in a technological sense) looks much different than just two or three years ago. Big data analytics is now intertwined with emerging technologies that give it extra power, accuracy, and quality.
Cloud computing, advanced databases, the Internet of Things (IoT), and blockchain are only some of the technologies that shape big data analytics and turn it into a powerful giant.
Advancements in Machine Learning and Artificial Intelligence
Machines may not replace us (at least not yet), but it’s impossible to deny their potential in many industries, including big data analytics. Machine learning and artificial intelligence allow for analyzing huge amounts of data in a short timeframe.
Machines can “learn” from their own experience and use this knowledge to make more accurate predictions. They can pinpoint unique patterns in piles of information and estimate what will happen next.
New Applications and Industries Adopting Big Data Analytics
One of the best characteristics of big data analytics is its versatility and flexibility. Accordingly, many industries use big data analytics to improve their processes and achieve goals using reliable information.
Every day, big data analytics finds “new homes” in different branches and niches. From entertainment and medicine to gambling and architecture, it’s impossible to ignore the importance of big data and the insights it can offer.
These days, we recognize the rise of big data analytics in education (personalized learning) and agriculture (environmental monitoring).
Workforce Development and Education in Big Data Analytics
Analyzing big data is impossible without the workforce capable of “translating” the results and adopting emerging technologies. As big data analytics continues to develop, it’s vital not to forget about the cog in the wheel that holds everything together: trained personnel. As technology evolves, specialists need to continue their education (through training and certification programs) to stay current and reap the many benefits of big data analytics.
Turn Data to Your Advantage
Whatever industry you’re in, you probably have goals you want to achieve. Naturally, you want to achieve them as soon as possible and enjoy the best results. Instead of spending hours and hours going through piles of information, you can use big data analytics as a shortcut. Different types of big data technologies can help you improve efficiency, analyze risks, create targeted promotions, attract an audience, and, ultimately, increase revenue.
While big data offers many benefits, it’s also important to be aware of the potential risks, including privacy concerns and data quality.
Since the industry is changing (faster than many anticipated), you should stay informed and engaged if you want to enjoy its advantages.
Have questions?
Visit our FAQ page or get in touch with us!
Write us at +39 335 576 0263
Get in touch at hello@opit.com
Talk to one of our Study Advisors
We are international
We can speak in: