The Magazine
👩‍💻 Welcome to OPIT’s blog! You will find relevant news on the education and computer science industry.
Search inside The Magazine
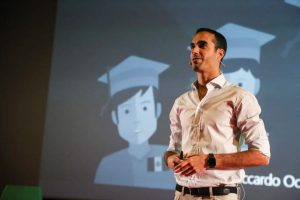
In this unite.ai article, Riccardo Ocleppo, Founder & Director of the Open Institute of Technology (OPIT), leverages his experience from Docsity to revolutionize higher education. OPIT aims to innovate and modernize education by offering high-quality tech degrees at an affordable price. It prioritizes hands-on learning to bridge the theory-practice gap and fosters diverse skill sets and networking opportunities.
Discover OPIT’s innovative approach, which includes embedding AI across all its degree programs, in the full article at unite.ai: https://www.unite.ai/riccardo-ocleppo-founder-director-of-opit-interview-series/
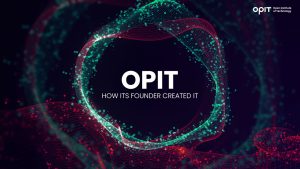
The Open Institute of Technology (OPIT) is a unique institution through and through. From an unparalleled support team that guides you every step of the way to state-of-the-art virtual resources, OPIT redefines online learning. This institution also proves that online education can be as enriching as its traditional counterpart. Better yet, it can outperform it in numerous aspects.
This fact alone begs the question – how did it all begin?
To answer this question, we’ll go straight to the source – the founder of OPIT, Riccardo Ocleppo.
In this article, Riccardo will walk us through his journey of envisioning (and building) OPIT and transforming online education in the process.
The Pre-OPIT Years: Where It All Began
To understand how Riccardo came up with the idea for OPIT, we must travel back to the year 2006. That’s when Riccardo graduated from Politecnico di Torino with a bachelor’s in electronics engineering.
This institution is arguably the most prestigious in Italy (and one of the most reputable in Europe). So, it shouldn’t be surprising that Riccardo chose to continue his education here, pursuing a master’s degree.
He completed the master’s program in 2008 and did so with honors.
Yet, Riccardo couldn’t shake the impression that it was all in vain. In his words, “When I left the university, I had the impression that I could do very little, and I knew very little that could help me in my professional endeavors.”
But Riccardo decided not to sit idly by.
He saw it this way – it might be too late for him, as he was done with his studies. But it’s certainly not too late for future students who deserve a better education. That’s why, only two years later, in 2010, he founded Docsity.
Docsity is an online social learning network with over 20 million registered students. Thanks to this network, over 250 universities worldwide received help in improving their study programs (and finding students).
Docsity also gave Riccardo a chance to fully immerse himself into the education sector for over a decade, finding new ways to reform it from within.
OPIT’s Inception: From Vision to Reality
With the knowledge (and the resources) from Docsity, Riccardo started working on a platform designed to provide the kind of education he wished he had received. The platform in question was, of course, the Open Institute of Technology.
The primary goal of OPIT was to bridge the gap between “what students expect, what companies need, and what higher-level institutions actually deliver in terms of training and education.”
From Riccardo’s experience, this gap was pretty huge. Remember that even with a bachelor’s and a master’s degree in electronics engineering, he felt he had little to offer to companies.
This perceived shortcoming primarily comes from the fact he received a lot of theory at the university but very little practice. And that’s not to mention how outdated the curriculum was, as well as laser-focused on electronics engineering. In other words, bid farewell to “competencies on the most recent technologies and project management methodologies.”
This perspective made him determined to create a holistic educational solution. Or, as Riccardo puts it, “When designing OPIT’s degree programs to address the skills in high demand today, we chose to start from scratch to go beyond the limits of traditional higher education.”
At OPIT, you’ll receive valuable knowledge beyond theory. Essentially, OPIT equips you with everything you need to enter the job market, ready to excel in your field from day one (or day zero, as Riccardo calls it!).
Tailored for Triumph: OPIT’s Unique Programs
Designing any online curriculum is no easy task. However, the computer science field comes with its unique set of challenges. Why?
This field is constantly evolving. That’s what makes it difficult for most traditional higher education institutions to keep up. As Riccardo puts it, “[These institutions] are very slow to adapt to this wave of new technologies and new trends within the educational sector.” Of course, thanks to Docsity, Riccardo speaks from extensive experience, as he’s seen “multiple times how difficult it is to help these institutions update their study curriculum.”
Companies have it no easier.
Riccardo says, “A company needs one to two years to make people that should be trained on today’s technologies and on today’s skills effectively enter the job market and be productive when they enter these companies.”
Again, Riccardo speaks from personal experience. As a founder of a tech company (and a manager in others), he was tasked with creating and managing big tech teams on several occasions. However, despite interviewing hundreds of candidates, he couldn’t find those trained in today’s technologies, not those from 20 years ago.
With this in mind, he designed OPIT’s curriculum to effectively “train the next generation of leaders and managers in the field of computer science.” Many people helped him in this endeavor, chief among them Professor Francesco Profumo, current head of institution at OPIT and former Minister of Education in Italy.
This unique approach makes OPIT’s programs different in terms of how they’ve been conceived and how they’ll be delivered.
Take the Bachelor of Science (BSc) in Modern Computer Science program as an example.
Riccardo says that to be a great programmer, “you cannot just dive into programming itself.” First, you must understand how a computer is built and how its various units operate and communicate. This way, you’ll have no issues debugging a code in the future since you’ll understand the underlying mechanisms.
These underlying systems and foundational skills are precisely what is taught during the first term of the modern computer science program. Afterward, you’ll move toward the latest advancements in computer science, including machine learning, artificial intelligence, data science, cybersecurity, and cloud computing. This way, you’ll have quite a broad perspective on computer science, rarely seen in other educational programs, online or offline.
It also means you won’t have to specialize in a particular field, as you’re forced to do with many other programs. In Riccardo’s opinion, the master’s degree is where you should begin your specialization journey.
OPIT offers as many as four master’s degree programs, but Riccardo focuses on Applied Data Science & AI this time.
In Riccardo’s words, “The whole purpose of this [program] is actually to train people that do not want to pursue a super technical career but actually want to pursue a career at the intersection between the tech and the management of a company.” In other words, individuals who complete this program will acquire all the necessary tech skills. However, they’ll also be able to ensure the tech team is “correctly understood by the management of the company,” thanks to the managerial skills earned during the program.
Of course, this program also covers all the essential theoretical knowledge, from Python to machine learning. But it also has a solid applicative angle, teaching students how to use the most valuable tools available in today’s market. Simply put, you’re training “for what you’ll be doing when you enter your next job.”
Breaking the Mold: What Sets OPIT Apart
The unique curriculum isn’t the only thing that sets OPIT apart from other higher education institutions in the same field. Here’s what Riccardo singles out as OPIT’s most appealing characteristics.
Continuous Assessment
Learning at your own pace can be a double-edged sword. On the one hand, you have all the flexibility and freedom to organize your studies (and life). On the other, you might start procrastinating without a traditional daily commitment of in-classroom learning.
OPIT ensures this unfortunate scenario never happens by doing away with one big final exam you must cram for. Instead, you’ll be continuously assessed throughout the program, allowing you a much better approach to learning and a deeper understanding of the subject matter.
As Riccardo puts it, OPIT will give you “multiple checkpoints,” preventing you from getting “lost” throughout the learning process.
New Learning Resources
According to Riccardo, most of today’s available resources were created for the “oldest wave of education.” That’s why he (and his team) created all OPIT resources and learning materials from scratch, giving you a fresh perspective on the tech world. These resources also come in the form of engaging videos, which are short enough to keep you fully focused yet detailed enough to provide a deep understanding of the topic.
World-Class Professors
Let’s not sugarcoat it – modern resources mean nothing if the professors teaching them still stick to old-school principles and approaches. Luckily, this isn’t the case with OPIT’s faculty.
Every member of this faculty has been carefully selected based on their academic expertise, business experience, and global perspective. These professors aim to “help you learn in a more engaging and interesting way,” as Riccardo puts it.
He also adds that OPIT’s faculty breaks away from the common saying in academics, “Those who can’t do, teach.” In his words, “We didn’t want to have people that can teach because they cannot do,” so that’s the standard he prioritized when bringing people on board.
Future-Proof Your Career
Now that you know the fascinating tale of OPIT’s conception, all that’s left to do is to get in touch with our team of experts and take the first step in future-proofing your career. As you’ve already seen, OPIT will take care of most of the subsequent steps. All you need is a desire to learn and an interest in developing new skills, and success is imminent.
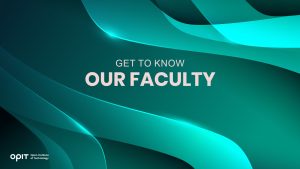
At the Open Institute of Technology (OPIT), we have a simple goal – to provide high-quality yet accessible education in the technology field. But for our courses to be high-quality, the professors behind them must be equally exceptional.
And they absolutely are.
The OPIT professors are paragons of expertise and passion. Each professor has been handpicked for their profound understanding of technology, coupled with extensive academic achievements and industry experience. That’s why these architects of knowledge bring both theoretical depth and real-world insights into every class at OPIT.
So, what better way to get to know our world-class OPIT faculty than to hear their thoughts on the topics they’re passionate about? In this article, you’ll read what five of our top-notch faculty members have to say about tech innovations transforming the world. Of course, you’ll also get a quick overview of other members of our inspiring faculty shaping the next generation of leaders in technology and digital space.
The OPIT Faculty at a Glance
Before diving into our faculty members’ fascinating biographies and insights, let’s take a moment to appreciate the diverse expertise that forms the backbone of OPIT.
Our faculty is a mosaic of over 30 accomplished professionals from all over the world, each bringing a unique perspective to the table.
And that’s the beauty of online learning. How else would you be able to connect with experts spanning the globe, all from the comfort of your own home?
Our inspiring faculty comes from over 15 countries and four continents. The U.S., Canada, Brazil, Lebanon, Germany, France, India and Italy are just some of the nations represented. This collage of diverse backgrounds (and experiences) ensures that your education at OPIT transcends geographical boundaries, offering a truly global perspective on technology.
Meet the OPIT Faculty at the Forefront of Technology
Now that you have a better idea of the diverse expertise within our faculty, let’s introduce you to the brilliant minds at the forefront of technology education at OPIT. This time, we asked five of our esteemed faculty members how recent tech innovations have transformed the world. Here are their answers.
Raj Dasgupta, Ph.D.
Professor Raj Dasgupta is an impressive individual in every regard. He’s currently a research scientist at the U.S. Naval Research Laboratory after teaching computer science for almost 18 years at the University of Nebraska. His research projects have been funded by the U.S. Department of Defense and NASA, and he has earned a Ph.D. in Computer Engineering from the University of California. Talk about a multifaceted professional!
At OPIT, Professor Dasgupta teaches Data Structures and Algorithms, Reinforcement Learning, and Introduction to Artificial Intelligence in the Bachelor of Science in Modern Computer Science program. The last subject is also a part of the Bachelor of Science in Digital Business program. He also teaches Machine Learning in the Responsible Artificial Intelligence program.
When asked about the transformative impact of tech innovations, Professor Dasgupta singled out the brain-computer interface (BCI) system as the technology that fascinates him the most. He explains, “We have been able to link the human thought, the human brain, with these assistive devices.” This connection means that these BCI systems can extract (and use) any thoughts from people who can’t speak for themselves or express their thoughts. As Professor Dasgupta puts it, all it takes is for them to “just think what they want to do.”
Santhosh Suresh, Ph.D.
With giants like PayPal, Meta, and McKinsey & Company on his resume at a young age, it’s evident that Professor Santhosh Suresh possesses remarkable expertise in business problem-solving. Business Problem Solving is precisely the subject he teaches at OPIT’s Master of Science in Applied Data Science & AI and Applied Digital Business programs.
So, it’s no wonder his answer to our question also focuses on solving problems, this time with technology. He rejoices at the fact that the ultimate knowledge is no longer reserved only for the rich and privileged. Thanks to advanced data science-based algorithms, “the efficiency of airlines or railroads or how we do operations in the surgery room has gone up exponentially, and that is improving the quality of lives of millions if not billions of people.”
Paco Awissi, MBA
A data science leader. An analytics expert. A machine learning practitioner. These are just some of the impressive attributes that define Professor Paco Awissi’s career. These flattering attributes also landed him the coveted positions of Vice President of Data and Reporting at Morgan Stanley, Lead Instructor at McGill University School of Continuing Studies, and, of course, Professor at OPIT.
Professor Awissi teaches three courses in our Master of Science in Applied Data Science & AI program – Project Management, Applications in Data Science and Artificial Intelligence (Part 2), and Business Communication.
When asked about new tech advancements, he also focuses on AI, explaining that the technology is revolutionizing “risk management, fraud detection, and personalized financial services.” Professor Awissi adds that AI is also used in “algorithmic trading, credit scoring, and automating customer service through chatbots, which improves the efficiency and inclusiveness of financial services.”
Filip Biały, Ph.D.
Professor Filip Biały comes from Poland, where he has taught at the Adam Mickiewicz University in Poznan for over 15 years. When it comes to Professor Biały, it’s hard to tell whether he has more education or experience in computer science and artificial intelligence.
However, his main goal is to understand the consequences of AI for democratic politics, which is why he also emphasizes that the negative impact of digital technologies shouldn’t be overlooked. As for the positive sides of this life-changing technology, Professor Biały says that it is “essential in improving the efficiency of business processes and advancing research, for example, in discovering new drugs.”
At OPIT, you can listen to Professor Biały’s fascinating insights in the Bachelor of Science in Modern Computer Science and Digital Business programs (ICT Fundamentals, Web Development, and Ethics of Computer Science & AI courses).
Tom Vazdar, Ph.D.
Like his colleague, Professor Tom Vazdar also primarily focuses on the negative implications of technological advancements. As a current AI and Cybersecurity Strategist of a boutique consulting firm and the former Chief Security Officer at Erste Bank Croatia, he knows just how important cybersecurity is and how dangerous technological progress can be without adequate safeguards.
That’s why he’s the expert OPIT put in charge of its latest Master of Science program – Enterprise Security. OPIT has worked closely with Professor Vazdar to develop this program and equip students with the most in-demand technical, managerial, and soft skills.
Professor Vazdar also teaches Introduction to Computer Security in the Modern Computer Science and Digital Business programs, as well as Behavioral Cybersecurity in the abovementioned Enterprise
Security program.
Meet More OPIT Faculty Members Helping You Succeed
If you apply to OPIT, you’ll get the unique chance to learn from the very best from all over the world. But until then, you can hear more intriguing perspectives from our faculty members. Visit the Faculty section of our website to get a sneak peek of the incredible expertise and global perspectives that shape OPIT.
The professors at OPIT have either taught at prestigious universities or have a long and impressive history in the industry. For the former, our professor’s biographies are adorned with institutions like the University of Copenhagen, the University of Rome, the Italian Institute of Technology, and the University of Stuttgart.
As for the latter, Microsoft, Meta, Symantec, and UBS are just some of the world-famous companies where our faculty members have left a lasting impact. The same goes for institutions like the Europol, the European Parliament, and the European Investment Bank (EIB).
Though our faculty members come from different corners of the world, they all share a common goal – a relentless pursuit of knowledge. By learning from these top-notch professionals, you’ll get an insight into decades of cutting-edge research, industry collaboration, and real-world experience. This knowledge and the skills you acquire at OPIT will help you play a leading role in the technological revolution, just like your professors.
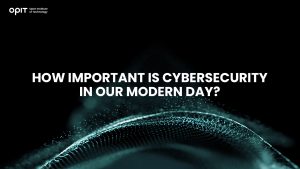
In the digital age, virtually every aspect of people’s lives is connected through digital channels. On the positive side, this allows instant communication and information access, as well as global connectivity. But this connectivity also introduces a myriad of risks, with cybersecurity threats chief among them.
In such an environment, protecting sensitive information and critical infrastructure has never been more crucial. And yet, the cybersecurity industry is short 4 million workers.
That’s why we invited Tom Vazdar, the program chair of the Master in Enterprise Cybersecurity program at the Open Institute of Technology (OPIT), to shed light on cybersecurity’s critical role in safeguarding our interconnected world. Professor Vazdar will also walk us through the Enterprise Cybersecurity Master’s program at OPIT, explaining what makes it stand out among similar programs.
With extensive experience in various industries (like finance and manufacturing) and countless successful cybersecurity strategies, risk management frameworks, and compliance initiatives under his belt, Professor Vazdar is truly the one to consult. His take on the pressing challenges (and solutions) within the cybersecurity field is invaluable for future students and those already in the industry.
The Current State of Cybersecurity
As Professor Vazdar puts it, “We are living in an era where digital transformation is accelerating.” So, it’s not surprising that new trends (and challenges) continue to emerge in the field. Here’s what Professor Vazdar has to say about them.
Cyberattacks Are Increasing
According to the ISACA’s 2023 State of Cybersecurity report, 48% of organizations reported an increase in cyberattacks compared to the year prior. Professor Vazdar says that this primarily has to do with the increasing complexity of cyberthreats. Simply put, organizations can’t keep up with the escalating sophistication of these threats, resulting in their increased frequency.
But there’s another element to this alarming increase in the number of cyberattacks – a lack of transparency. You see, Professor Vazdar claims that many organizations are believed to underreport cyberattacks. Such underreporting might be due to concerns about reputational damage or regulatory consequences. Either way, it’s exceptionally harmful to the industry, as it hinders the ability to collaborate on developing effective countermeasures and strengthening collective cybersecurity defenses.
Cybersecurity Lacks Workers
As previously mentioned, the cybersecurity industry is experiencing a severe staffing challenge. Interestingly, this doesn’t mean the number of cybersecurity professionals is decreasing. It’s quite the opposite, really.
In 2023, the global cybersecurity workforce grew 8.7% to reach 5.5 million people, a record high. And yet, another 4 million professionals are needed to meet the escalating demand for cybersecurity. If there has ever been a stat to prove just how critical cybersecurity is, this undoubtedly does it.
New Technologies Are Constantly Adopted
Artificial intelligence. Machine learning. Cloud computing. Internet of Things. Blockchain technology. These are just some of the technologies Professor Vazdar singles out as transformative forces reshaping cybersecurity.
On the one hand, these technologies have the power to enhance threat detection and cybersecurity response. On the other, they can also introduce new vulnerabilities and threats, such as data poisoning. The worst part? We’ll let Professor Vazdar explain it:
“All of this has come in a really short period of time, and we, as people, are actually struggling to learn about all these new technologies.”
That’s why he emphasizes the need for continual education in the field, as this is the only way to stay ahead of the curve.
Cybersecurity Strategies Are Becoming Proactive and Predictive
Here’s how it used to be in the cybersecurity world, according to Professor Vazdar: A new massive threat would emerge every few years, affecting the whole world. In the aftermath, you would scramble a team together and work tirelessly for a few days to develop a patch or a solution.
As you can imagine, this approach is hardly viable in today’s oversaturated cybersecurity landscape. That’s why “we’re seeing a shift toward more proactive and more predictive security strategies,” as Professor Vazdar puts it.
Cyberpsychology Is Gaining Importance
Cyberpsychology is by no means a new concept. According to Professor Vazdar, this term was first used in 2008 by Professor Zheng Yan. However, its significance has grown exponentially in recent years. This field of study shifts the focus from the cyberthreat to the cyberattacker.
Its goal is to understand what these malicious actors are doing and why. The result? “We, as humans, know how to defend [ourselves].”
According to Professor Vazdar, this is the third (and most important) layer of defense against cyberthreats. The first concerns the physical environment (i.e., the computer and information systems), while the second is a logical layer that “connects everything together.”
No One Is Immune to Cyberthreats
There’s a common misconception that smaller organizations and individuals aren’t “appealing” to hackers and other malicious actors. However, this couldn’t be further from the truth. No one is immune to cyberthreats, as cybercriminals always have something to gain (regardless of the target’s size or perceived importance).
That’s why investing in cybersecurity is crucial, whether you work for a small IT team or a huge company or just use technology in your day-to-day life.
Why Continuous Education Matters in Cybersecurity
There’s no doubt about it – cybersecurity should be a top priority for everyone in the industry and beyond. But as Professor Vazdar has underscored, what was effective in cybersecurity yesterday might not be sufficient today.
That’s why he emphasizes that “it’s important to get educated [now] more than ever.”
After all, there’s a single constant in the ever-changing cybersecurity field – humans as a crucial line of defense. The more people get educated, the more resilient the protection against cyberthreats becomes.
Why Pursue a Master’s Degree in Cybersecurity at OPIT
One of the postgraduate programs offered by OPIT is the Master of Science (MSc) in Enterprise Cybersecurity. This program is fully remote and can be completed in 12 to 18 months. But enough with the logistics – what makes this program the right choice for getting the much-needed education mentioned above?
Given that he practically shaped this program, Professor Vazdar is the best person to ask this question. He shares with us what makes this program uniquely positioned to prepare students for all the cybersecurity challenges he has touched on in this article.
A Comprehensive Curriculum
According to Professor Vazdar, the first thing that sets this program apart is “the curriculum depth and breadth.” This program covers various topics, from cybersecurity fundamentals (the first module) to advanced areas like AI-driven cybersecurity (the second module).
In other words, this program guarantees two things – a solid cybersecurity foundation and a deep dive into specialized topics. This focus makes it ideal for individuals seeking a well-rounded education in corporate cybersecurity, regardless of their previous experience in the field.
A Unique Structure
Unlike most programs in the industry, OPIT’s Enterprise Cybersecurity program doesn’t solely focus on the technical aspects of cybersecurity. But it doesn’t only dive into the managerial aspect of it either. Instead, it gives you just the “right blend of knowledge,” as Professor Vazdar puts it. Thanks to this approach, you can start working immediately after completing the program. After all, you’re all set skill-wise!
Alignment With Industry Certifications
Industry-standard certifications are becoming increasingly important, as most employers prioritize them when hiring new people. If you’re considering a career in cybersecurity, you’ll be happy to know that OPIT’s Enterprise Cybersecurity program is fully aligned with industry certifications like the Certified Information Systems Security Professional (CISSP). As Professor Vazdar puts it, this ensures that OPIT graduates are “not only academically proficient but that they’re also industry-ready.”
It’s also important to note that this program is internationally recognized and ECTS-accredited by the European Agency for Higher Education and Accreditation.
An Emphasis on Practical Applications
The Enterprise Cybersecurity program places a strong emphasis on practical applications. After all, this is the only way for OPIT students to be industry-ready upon graduating. That’s why the entire third module of the program is dedicated to a Capstone project, a hands-on endeavor that also serves as your dissertation.
A Supportive Environment
One of the aspects of studying at OPIT we’re most proud of is our carefully crafted support team. From the class coordinator to the career advisors, everyone at OPIT has a single goal – to help you succeed.
To this end, all the professors in the Enterprise Cybersecurity program (and beyond) are either academics or experienced professionals with plenty of valuable insights “from the forefront of cybersecurity.”
This course includes interactive lessons, live lectures, and private mentoring sessions, ensuring you never feel alone or isolated at OPIT.
Unparalleled Flexibility
One of the primary reasons for choosing online studying is its incredible flexibility. But OPIT takes this aspect to another level. Besides dictating your own study pace, OPIT lets you choose from several elective courses, allowing you to tailor your learning to your interests and career goals. Professor Vazdar singles out the following courses as the most appealing in terms of what this article has discussed:
- Behavioral Cybersecurity
- Secure Software Development
- AI-Driven Forensic Analysis in Cybersecurity
Give Yourself a Competitive Edge With OPIT
OPIT’s Master of Science in Enterprise Cybersecurity program does much more than educate students. It also prepares them for the future, allowing them to become leaders in cybersecurity. As Professor Vazdar puts it, “Our graduate students will be well-equipped to tackle current and future cybersecurity challenges in different sectors.” And given just how quickly these challenges evolve, you can’t really put a price on such preparation (and education).
So, get in touch with our team of experts to give yourself a competitive edge in the dynamic field of cybersecurity.
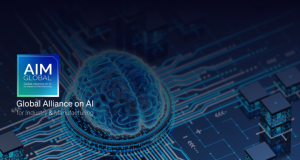
Malta – 19 January 2024  – OPIT Open Institute of Technology has joined AIM Global, the Global Alliance on Artificial Intelligence for Industry and Manufacturing. This strategic collaboration reflects our commitment to advancing safe, sustainable, and inclusive use of AI in industry and manufacturing.Â
Guided by the United Nations Industrial Development Organization (UNIDO), AIM Global promotes the responsible development and deployment of AI and frontier technologies. Aligned with the UN Secretary-General’s Our Common Agenda, AIM Global shapes a digital future that is open, secure, and beneficial to all.
AI technologies are transforming industry and manufacturing, offering unprecedented opportunities for increased efficiency, productivity, and sustainability. However, the adoption of these technologies also brings ethical considerations, privacy concerns, and the imperative for inclusivity. As a new member, OPIT is excited to contribute to AIM Global’s mission of unlocking the full potential of AI for industry.
In the intersection of tech and education, OPIT is a leader, spearheading advancements in Artificial Intelligence (AI). Renowned for shaping future leaders, OPIT incorporates the latest AI technology into its programs, setting a new standard for innovative education across diverse sectors, including manufacturing. By providing individuals with skills for AI-driven manufacturing, OPIT continues to lead in nurturing talent for the industries of tomorrow.Â
“OPIT Open Institute of Technology is excited to join AIM Global, where forward-thinking partners collaborate to harness the power of AI responsibly,” said Riccardo Ocleppo (Founder of OPIT). “By being part of this community, we anticipate collaborative opportunities, innovative solutions, and a shared vision for the ethical and effective application of AI to unfold during this exciting journey.”
For media inquiries or further information, contact hello@opit.comÂ
Â
About AIM GlobalÂ
AIM Global fosters collaboration for responsible AI in industry and manufacturing. The alliance focuses on research, skills development, ethical guidelines, and policy recommendations to address challenges and opportunities in the evolving AI landscape.
For those interested in learning more about AIM Global, please visit the website at: http://aim.unido.org
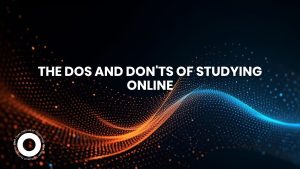
Adjusting to studying online is no easy task, especially if you’re used to a traditional classroom setting. But once you find your rhythm, you’ll realize that no traditional classroom can match the flexibility, convenience, and accessibility of online learning.
To help ease your success, Rosario Maccarrone, the Head of Student Services at the Open Institute of Technology, has compiled a detailed list of dos and don’ts of studying online. Stick to these guidelines, and you’ll excel at your studies as a full-time student or a working professional.
The Dos and Don’ts of Studying Online for Full-Time Students
Let’s say you’ve decided to commit to full-time online studies. Here’s what Rosario suggests for academic success.
The Dos
1. Find a Study Rhythm That Works for You
The best thing about studying online is that no one’s around to dictate your study schedule. It’s up to you to set your own learning pace and study hours. Find a steady study rhythm that aligns with your preferences, and your productivity will never falter.
2. Make a Schedule
Don’t get it twisted – a lack of a fixed class schedule doesn’t mean you should go without a schedule entirely. Keeping a schedule will help you stay organized, focused, and on track with your studies, which can be challenging when no one guides your daily routine. Your schedule should allocate time for classes (OPIT offers one live class per week for each course), revising, and, of course, taking it easy.
3. Keep a To-Do List
Just like a schedule, a to-do list can help you always stay on top of your game. Make it digital, and you can easily update it as you study or complete tasks on your computer.
4. Don’t Be Shy to Ask Questions
It’s perfectly OK to struggle with some parts of your online courses. However, it’s not OK to keep this to yourself out of shame or fear and struggle in silence. Your professors and tutors are there for you to guide you every step of the way. Remember this, and you’ll have no trouble asking questions when things get tricky.
5. Use All the Online Resources Your Program Offers
Just like your professors are there for you, so are the abundant online resources your university offers. Do a little research to get familiar with these resources and then make the most of them.
6. Collaborate With Your Classmates
You might not be able to see your peers in person, but you can—and should—communicate with them in a myriad of ways. Rosario suggests participating in chats during live sessions and taking advantage of every chance for group work. This will help you build a solid online community and nail your studies.
7. Join in on Online Campus Events
Studying is never just about learning. It’s also about meeting new people, making friends, and building a network. Online studying is no different; you’ll just do all of these incredible things online. So, make sure to keep up with your university’s virtual campus events and attend them to keep your studies (and life) more interesting.
8. Make a Study Spot
Let’s not sugarcoat it – learning from home often comes with a bunch of distractions. From your family talking in the background to the allure of your comfy bed, distractions are plenty. By making a designated study spot somewhere quiet, you’ll rid your study environment of disruptions and signal to your brain that it’s time to get down to work.
9. Take Care of Your Health
Taking care of your health is essential for excelling in all areas of life, and (online) studying is no different. So, remember to eat well, exercise, and get enough sleep to stay sharp. Exercising (or just moving continuously) is especially important given the nature of online studies.
The Don’ts
1. Procrastinate
Telling you not to procrastinate when studying is by no means revolutionary advice. However, avoiding procrastination can be extra challenging when studying online, given the lack of immediate supervision.
But putting off practice and leaving studying to the last second can only increase your anxiety levels and lead to lower grades (none of which will help you have a successful online studying experience).
2. Underestimate the Effort Online Classes Need
Some students operate under the assumption that online classes are less demanding than traditional ones. However, this couldn’t be further from the truth. Treating online classes with the same seriousness as in-person ones is the only way to succeed in your virtual academic journey.
3. Cut Yourself Off From Classmates and Professors
Online studying can feel rather isolating. That is – only if you let it. Online universities like OPIT offer numerous ways to stay in touch with your classmates and professors (e.g., Slack, Canvas discussions, and WhatsApp groups), and that’s precisely what you should do.
4. Hold Back From Asking for Help
This guide has already covered the importance of asking your professors questions. But if you feel stuck, don’t limit yourself to professors only. At OPIT, you can also ask your class coordinator or a tutor for help with anything course-related.
5. Neglect Taking Time Out for Yourself
Some students underestimate the effort necessary for online classes. At the same time, others go all out when presented with the seemingly endless possibilities of online learning. If you fall under the second category, don’t forget to take time out for yourself. This is as important as studying efficiently, as it allows you to prevent burnout.
6. Ignore Your Mental Health
Your mental health is just as important as your physical well-being. If you struggle emotionally, you should never ignore it. Your friends, family, or your university’s counseling services can help you find the best way forward. But first, you need to ask for help.
7. Miss Out on Making Connections With Peers
The beauty of online studying is the opportunity to meet new people from all over the world. OPIT alone has students from over 40 countries worldwide. Connect with them, and you’ll broaden your perspective while potentially forming meaningful connections.
Of course, you should also enjoy face-to-face interactions whenever possible.
The Dos and Don’ts of Studying Online for Working Students
Thanks to online studies, it’s never too late to go back to school. You can do so even after working for a few years, as this format allows you to pursue a new career path or enhance your skills while still earning an income.
Of course, you can also choose to work and study at the same time immediately after graduating from high school.
Whatever the case, here’s what Rosario suggests doing (and not doing).
The Dos
1. Balance Your Work and Study Time
When you have both work and studies, you must allocate your time wisely. Creating a realistic schedule that you can keep up with daily is step one.
2. Talk to Your Boss About Supporting Your Study Goals
Most bosses are highly supportive of their employees’ professional development. After all, your personal growth can only positively reflect on your company. So, don’t be afraid to talk to your boss about your studies.
With some luck, you’ll be able to schedule shifts around your classes, take days off for studying (or taking exams), and even study at work during downtime.
3. Strive for Flexibility at Work
If there’s one thing the COVID-19 pandemic has taught us, it’s that most jobs can be done from home (and done successfully). So, if possible, switch to working from home, as this will save you a lot of time. Just think of it this way – no more getting ready and commuting to and from work.
4. Connect With Colleagues in Similar Situations
Balancing work and studies can sometimes feel overwhelming. Connect with colleagues who are also going through the same thing (or did in the past), and you’ll get all the encouragement you need to keep pushing forward.
5. Grab Chances to Build Skills for Work and School
Let’s face it – most people study to be able to find a decent job. If you’re already working in your desired field – perfect! Seize every chance to apply your practical skills in your studies. Even if you aren’t currently working in your desired field, there are always skills you can learn to benefit your studies and current (and future) career.
6. Set Realistic Study Goals
Doing (or trying to do) too much can bring you no good. So, set realistic study goals that align with your work life. For instance, studying for three hours a day will be a Herculean task when working overtime but a breeze on your day off.
7. Talk to Your Professors
Just like you should let your boss know you’re studying, you should also inform your professors that you’re working. This way, they might be able to make some accommodations for you if your work is squeezing your study time.
8. Keep Your Study Area Separate
Let’s say you work from home. If so, make sure you have separate “work” and “study” areas. This will help you maintain a clear boundary between your professional and academic responsibilities.
9. Find Ways to Manage Stress
Juggling work and studying can be rather stressful. Finding a way (or ways) to manage this stress is critical to successfully keeping up with both responsibilities. Meditating, exercising, taking a walk, spending more time with friends, or taking up a hobby are just some of the methods that might work for you.
Taking regular breaks is also an excellent way to manage stress as well as remain focused on your work or studies.
The Don’ts
1. Let School Stress Affect Your Work Performance
If you’re already working in your dream field, remember that studying is only there to enhance your skills and knowledge. In other words, don’t let academic stress affect your work performance, as this can only jeopardize your future in your dream career. It’s all about prioritizing correctly.
2. Sacrifice Sleep or Good Health Habits
Juggling work and studies is no excuse to fall into bad health habits, including sacrificing sleep. Not only will this affect you physically and mentally, but it will also affect your performance in both fields. Talk about a lose-lose scenario!
3. Be Afraid to Ask for Help
Your support network is all you need to navigate any challenge effortlessly. And with both working and studies, you know you’ll have no shortage of those. So, don’t be afraid to ask for help from the people around you, whether to simply listen to you vent or help with a specific task.
4. Let Your Studies Take Over Your Personal Time
For most people, off time is a chance to rest and recharge. However, for students, a part of this time must be dedicated to studying. But the key word here is “part.” Don’t let your studies consume all of your personal time, as this is a one-way ticket to burnout.
5. Miss Out on Networking in Your Field
If there’s one piece of advice every university (online or offline) will give you, it is network, network, network. If you’re already working in your field, you’re in a unique position to do this even before completing your studies. So, take every chance to meet new people and open up new doors for your career.
6. Neglect Seeking Mutual Work-Study Benefits
Your job and studies can likely benefit each other in more ways than one. By neglecting to explore these avenues, you might miss out on valuable opportunities.
For instance, let’s say you’re getting your Bachelor’s degree in Digital Business from OPIT and working in social media management. Modules like “Digital Marketing” and “Digital Platforms and Ecosystems” could directly contribute to your role (and a promotion). At the same time, your practical experience will help you breeze through most of the course material.
This is just one of the ways your studies can open new doors at your work.
7. Overcommit Socially
Your time is precious. As much as it’s important not to use it solely for work and studying, it’s also crucial to not overcommit socially. Talk to your friends and family (especially those with no similar experiences) and set realistic expectations about your availability. Prioritize quality over quantity in your social interactions, and you’ll never feel like you’re missing out.
The Biggest Do: Study at OPIT
As you’ll learn from this list, everyone at OPIT knows what our students need to succeed on and off campus. And everyone at OPIT, from Rosario to your professors, is more than eager to support you in this journey. Apply to study at OPIT and experience what quality studies, academic assistance, and career guidance can do for you firsthand.
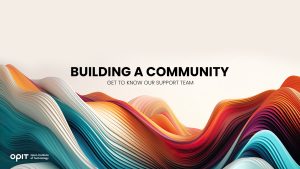
Although convenient, online studying can also feel isolating. After all, you’re all alone in front of a screen, probably missing the camaraderie and face-to-face interactions of a physical classroom.
That’s why our team at the Open Institute of Technology set out to change the typical online education experience, breaking down all the virtual barriers before them. The goal is clear – OPIT students are never to feel alone during any step of their academic journey. And this goal wasn’t only met but surpassed rather spectacularly (if we do say so ourselves).
Are you interested to know how our team pulled it off? Great! At OPIT, we love questions, as well as sharing success stories.
To answer this question thoroughly, we enlisted the help of Rosario Maccarrone, our Director and Head of Student Services, and Sara Ciabattoni, our Class Coordinator. They’ll break down OPIT’s impressive support system, which has effortlessly transformed students from over 40 countries worldwide into a close-knit community.
How Does OPIT Support Its Students?
When it comes to student support, one thing’s for sure – few (if any) remote learning programs offer such an intricate and well-thought-out web of support as OPIT does. This support starts before you even enroll in the Institute and often ends only after you’ve landed your dream job.
Admission Support
At OPIT, we have a dedicated admissions team that guides applicants every step of the way – from expressing interest in a specific course to becoming a student. This team has a simple goal – to ease your path into OPIT. So, don’t hesitate to ask any questions regarding the application process itself, program details, or course requirements.
Administrative Support
Let’s not sugarcoat it – starting a new program can be extremely chaotic. You might have trouble enrolling in courses, managing your schedule, and navigating all the complexities of a new academic environment. But don’t worry; it’s our administrative support team to the rescue! This team will help you breeze through all the administrative requirements, from applying for courses to getting certificates.
In this regard, we must also mention the lovely Sara, aka our class coordinator.
Class Coordinator
The class coordinator is here to help the OPIT students with their everyday needs and tasks. This might include questions about classes, deadlines, or interactions with other students and faculty members. Let’s put it this way – if you’re ever unsure who to reach out to, Sara is your go-to person.
As she puts it, “You can reach out to me, and I will guide you.” Take this as a blanket statement, whether you have a specific question or are simply struggling with time management or your studies.
Learning Support
At the end of the day, all OPIT students are here to learn. That’s why Rosario made it his mission to ensure top-notch learning support for each and every OPIT student.
Tutors
It’s perfectly natural to struggle with specific parts of your coursework, especially if you’re dealing with these concepts for the first time. That’s why there’s a dedicated team of tutors at your disposal at all times.
When we say “at all times,” we truly mean it! These tutors are available seven days a week, every day of the calendar year. You can reach them and ask for any lecture-related assistance you might need.
You’ll promptly receive an answer from these professionals who have strong backgrounds in computer science and tech. Most of our academic tutors have a PhD or are currently obtaining one, so you can rest assured you’re in good hands.
Professors
With classroom learning, posing a question to your professor is a breeze – you just raise your hand! In most online classrooms, however, this option doesn’t exist since classes are usually pre-recorded.
That’s why OPIT offers a live class once a week featuring a handy Q&A session. This session is the perfect opportunity to directly interact with professors in charge of different subjects, gather all the necessary information, or resolve any doubts. After all, some questions are quite complex and can only be answered by the experts.
And that’s precisely what our professors are. Some of them have taught in universities for their entire careers. Others have a long and impressive history in the industry, having worked at tech giants like Microsoft and Meta. Either way, our careful selection of professors is a guarantee that “what we say is what we do,” as Rosario puts it.
Continuous Assessment
Though this isn’t a team per se, the continuous assessment methodology employed by the OPIT faculty is a crucial element of support for our students.
This methodology is exactly what it sounds like – students are assessed continuously throughout the duration of their courses. There are many pedagogical benefits to this approach, but the two primary ones are as follows.
One, our students can advance their knowledge gradually, which allows them to retain and apply information more effectively. And two, it eliminates the stress of cramming for the big and scary final exam that often overwhelms the students. Sara emphasizes that this approach allows you to enter the job market with a well-rounded understanding of your field.
Career Support
Speaking of entering the job market, OPIT will lend you a helping hand in this regard as well. The goal of the career support team is to “help students shift from their academic journey into their professional journey [with ease],” as Sara puts it.
Career Advisors
Given that most OPIT programs equip you with skills for dozens of different career paths, you might struggle with choosing only one. That’s where our career advisors come into play.
As you approach the end of your academic journey, these advisors will work with you to assess your skills, capabilities, and qualities, helping you pinpoint the most suitable career path. Then, they’ll review your CV and coach you on presenting yourself to potential employers in the best possible way. They’ll even help you look for a job and present you with some tailored job listings.
Internship Opportunities
If you want to gather more practical experience before fully entering the professional arena, our career advisors can help you find tailored internship positions to suit your needs.
Practical Skills
There’s a reason the above section is so short. OPIT prides itself on its competence-based approach that lets students “learn by doing,” as Rosario puts it.
OPIT courses are pretty hands-on, with at least two assignments whose goal is to help you put your knowledge into practice. Rosario calls this “filling the gap between theoretical knowledge served by classical universities and applied knowledge that is more often than not given through boot camps or non-accredited courses.”
The goal of this approach is to ensure students can “hit the ground running” and “apply their knowledge from day one.” There’s no need to go through a lengthy training process or learn anything from scratch!
Communication Channels
Given that our mantra at OPIT is “You’re not alone,” it shouldn’t surprise you that we offer several ways to communicate with fellow students and faculty staff.
Canvas
Canvas, our learning platform, is a treasure trove of knowledge and valuable resources. However, it can also serve as a vibrant communication hub.
You see, Canvas offers an option to start (or join) discussions and ask questions regarding anything course-related. Sometimes, professors can even publish some interesting and thought-provoking prompts that go beyond the traditional course material.
In addition, during some live classes (hosted on Zoom and available in Canvas), students are divided into smaller groups and put into breakout rooms. This typically gives them 10 to 15 minutes to work together and start a little discussion or debate. Sara says that she sees “a lot of interaction there,” as she has access to all courses and can review the exchanged messages.
Slack
While Canvas is the ultimate learning platform, it’s slightly too formal for student interactions. That’s why we also provide our students with access to a Slack channel for their classes and each of their courses. Here, students can share “whatever they see fit,” as Rosario puts it.
Moreover, Slack is a valuable tool in the professional context (widely used and integrated into workflows in many companies) – by being able to know and use the tool, students get closer to their job market.
Other Communication Channels
OPIT students are free to use whatever communication channels they want. It’s only natural for them to want to communicate in an environment that’s entirely under their control, as opposed to faculty-controlled Canvas and Slack.
We see no problem with this. In fact, it’s quite the opposite! As Sara explains it perfectly, “We’re really proud because we’re building a community.”
For now, our students prefer communicating via WhatsApp groups and Discord servers, which they use to share their experiences, feelings, and problems. Rosario says that it’s easy to tell what some of these discussions are, as they often spill over in some of the requests the Institute receives in terms of administration and term organization. Of course, we’re always open to feedback and do our best to accommodate the preferences of our students, especially when they’re so wonderfully united.
At OPIT, You’re Never Alone
And there you have it – the complete support system devised to ensure that every OPIT student feels connected, supported, and empowered throughout their academic journey. Apply for one of our highly acclaimed programs today and experience this unparalleled support firsthand.
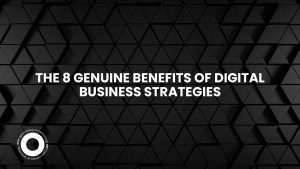
Before the COVID-19 pandemic, most businesses viewed digitalization as only an additional avenue for marketing their products or services. In the post-pandemic world though, digitalization has become the very lifeblood of survival for these businesses.
But implementing an efficient digital business strategy is no easy task. After all, a digital transformation calls for fundamentally reshaping how a business operates.
However, one look at what technology can do for you and your business is enough to make you understand that the complex digital transformation process is well worth it. If all of this is new to you, don’t worry. You can master all the necessary digital skills to reap the benefits of digitalization by completing the Open Institute of Technology’s Bachelor’s Degree in Digital Business. Alternatively, you can further your skills with the Institute’s Master’s Degree in Applied Digital Business.
Without further ado, let’s discuss eight genuine benefits of embracing digitalization and implementing digital business strategies.
1. Data-Driven Decision-Making
Efficiency. Innovation. Sustainable success. These desirable outcomes can only be reached with data-driven decision-making. While traditional decision-making mostly relies on experience, modern data-driven decision-making leverages the power of artificial intelligence (AI) to analyze vast amounts of data swiftly and accurately.
Of course, artificial intelligence brings much more to the corporate world beyond data analysis. This innovative technology can automate administrative tasks, provide instant customer responses, and enhance overall operational agility. All of these make managerial and business decisions and processes significantly faster.
That’s precisely why OPIT’s study programs feature courses dedicated to artificial intelligence in the business setting, from a general introduction to AI principles in the digital economy. The goal of these courses is simple – help you understand why, how, and when to use AI in business decision-making and beyond.
But as powerful as AI is, you can’t solely rely on technology in your decision-making process. AI is only there to help you make this process more comprehensive and strategized. This technology will also help you spend less time on mundane, control-based tasks, allowing you to focus on your judgment, critical thinking, and problem-solving.
Luckily, all of these skills are also covered by various Digital Business courses at OPIT, allowing you to become the best digital-era manager and decision-maker you can be.
2. Faster Time to Market
All the invaluable data gathered by artificial intelligence and other digital tools can also do wonders in the research and development department. With so much data at hand, businesses should have no trouble improving their existing products or services or launching new ones.
The latter process is especially important as the time it takes for a product to go from conceptualization to market availability can be rather lengthy. But what used to take months or years can now be done in weeks, days, or even hours, all thanks to digitalization.
Businesses can rely on modern technologies to rapidly test, pilot, iterate, and launch new products and services, significantly enhancing their agility in the ever-evolving business world. And hey, if a product or a service doesn’t quite work, you’ll be able to tell this almost immediately through online feedback and product reviews.
Using these valuable insights, businesses can revise their offerings to suit their customers’ preferences, needs, and interests. The result? Nothing but product excellence and customer satisfaction.
3. New Sales Channels
Dominating the business world is all about finding new ways to reach, engage, and retain customers. The good news? The digital-age business world offers seemingly endless opportunities to do this. You just need to know where to look.
Granted, your job doesn’t stop when a new channel is identified. Rather, it transforms. Now, it’s up to you to know how to use that channel effectively. To do so, you’ll need to learn about digital business models, as virtually every sales channel has a dedicated one.
Arguably, the most important thing to cover is how digital business models differ from traditional models (especially if you’ve only ever worked with the latter). And that’s precisely what OPIT’s “Digital Business Models” module covers.
You’ll learn just how vital online platforms, e-commerce, and digital marketing are in shaping modern business strategies. Use this knowledge to successfully tap into new markets and connect with a broader audience.
Naturally, there’s one online channel that’ll play critical role here – social media. Luckily for business owners and managers, most customers are active on at least one social media platform, essentially making the global population a prospect for sales.
4. Increased Market Share
For most businesses, the ultimate goal is to boost revenue, improve bargaining power, and strengthen competitive position. First step? Capturing a more substantial portion of the market. Fortunately, doing so is much easier now that most of the global population is within reach through digital business strategies.
So, it’s no wonder digital transformation has become essential to growing a brand. This process facilitates everything you need to establish a robust market presence – better financial performance, media activity, and advertising capabilities.
Best of all? You can use digital business strategies to only boost your market share or completely dominate your industry. It’s all up to you and how you harness the power of digitalization.
That’s why OPIT’s Applied Digital Business program contains a course titled “Maximizing Impact in the Digital Economy.” From gradual scaling to growth hacking, this course equips you with the insights and skills needed to ensure sustained success in virtually any industry.
5. Better Customer Interaction
Reaching and retaining new customers are equally important. But how can you do this? By ensuring most (if not all) of their interactions with the business are positive, of course. Naturally, digital transformation can help in this regard as well.
After all, the digital environment offers countless possibilities for improving the customer relationship with a brand. From streamlined online transactions to personalized communication through various digital channels, there’s virtually no area of the customer experience that digital transformation can’t positively impact. With this process, it’s all about simplicity, real-time services, and tailored experiences. And there’s hardly a customer that doesn’t love these things.
Granted, many (digital) elements are involved in achieving this desirable outcome, including artificial intelligence, automation, and digital marketing. But don’t worry; the OPIT’s programs extensively cover each element.
Take digital marketing as an example. Whether you’re interested in gaining an understanding of the key concepts of this dynamic field or delving into advanced strategies, there’s a module tailored to your needs.
6. Real-Time Transactions
As previously mentioned, customers love real-time services. In the past, this was hardly possible, as businesses were limited by time and infrastructure constraints. But not anymore. With digital tools, the 24/7 concept becomes a reality.
Customers can look at business offerings and make a purchase at any time of the day or night, breaking free from the traditional constraints of operating hours. All they need is a few clicks, and voilà – their transaction is complete.
This is made possible by innovative technologies like Blockchain, which dramatically enhances transaction speed while minimizing costs and any chances of fraud. Of course, this also means that this technology is covered by OPIT’s undergraduate program, which looks into the Blockchain’s transformative impact on business practices.
7. Easy Talent Acquisition
So far, this article has focused on how digital business strategies can benefit a brand’s relationship with customers. But these strategies can also revolutionize the process of talent acquisition. Of course, this is equally important, as there’s no successful business without a talented and capable workforce.
Before, recruiting top talent was often a challenging and time-consuming endeavor. Now, attracting talent (and the right talent for your business at that) is almost effortless. How?
Well, digital tools have got your back every step of the way. From job-matching sites like LinkedIn attracting candidates based on their professional profiles to automated systems streamlining the hiring process, digital tools have completely transformed talent acquisition.
That’s not to mention the endless opportunities working from home has opened up both for businesses and employees. Thanks to digitalization, the whole world is basically your talent pool.
8. Higher Revenue
Though each of the mentioned benefits is essential for business success in and of itself, they also have a cumulative impact on one of the most crucial business metrics: revenue. Investing in digitalization might seem like a major undertaking, but this impact makes every penny worth it.
Let’s recap what you can get with effective digital business strategies. You can get more effective systems and productive employees, better decision-making and judgment, improved products and sales channels, and a long-lasting (and interactive) relationship with customers. All of these create a robust foundation for revenue growth.
Reap the Full Benefits of Digital Business Strategies
There’s no doubt about it – effective digital business strategies can transform the trajectory of virtually any business. But for these strategies to work, they must be carried out with precision, knowledge, and strategic foresight.
That’s where OPIT’s Bachelor’s Degree in Digital Business and Master’s Degree in Applied Digital Business come into play.
Regardless of the digital business career you’re interested in (e.g., digital business consultant, digital marketing specialist, or digital transformation specialist), these courses will equip you with the necessary skills to stay ahead in a globally connected world.
Have questions?
Visit our FAQ page or get in touch with us!
Write us at +39 335 576 0263
Get in touch at hello@opit.com
Talk to one of our Study Advisors
We are international
We can speak in: